DiverSim: an Inclusive Simulator for Diverse Synthetic Pedestrian Data Generation
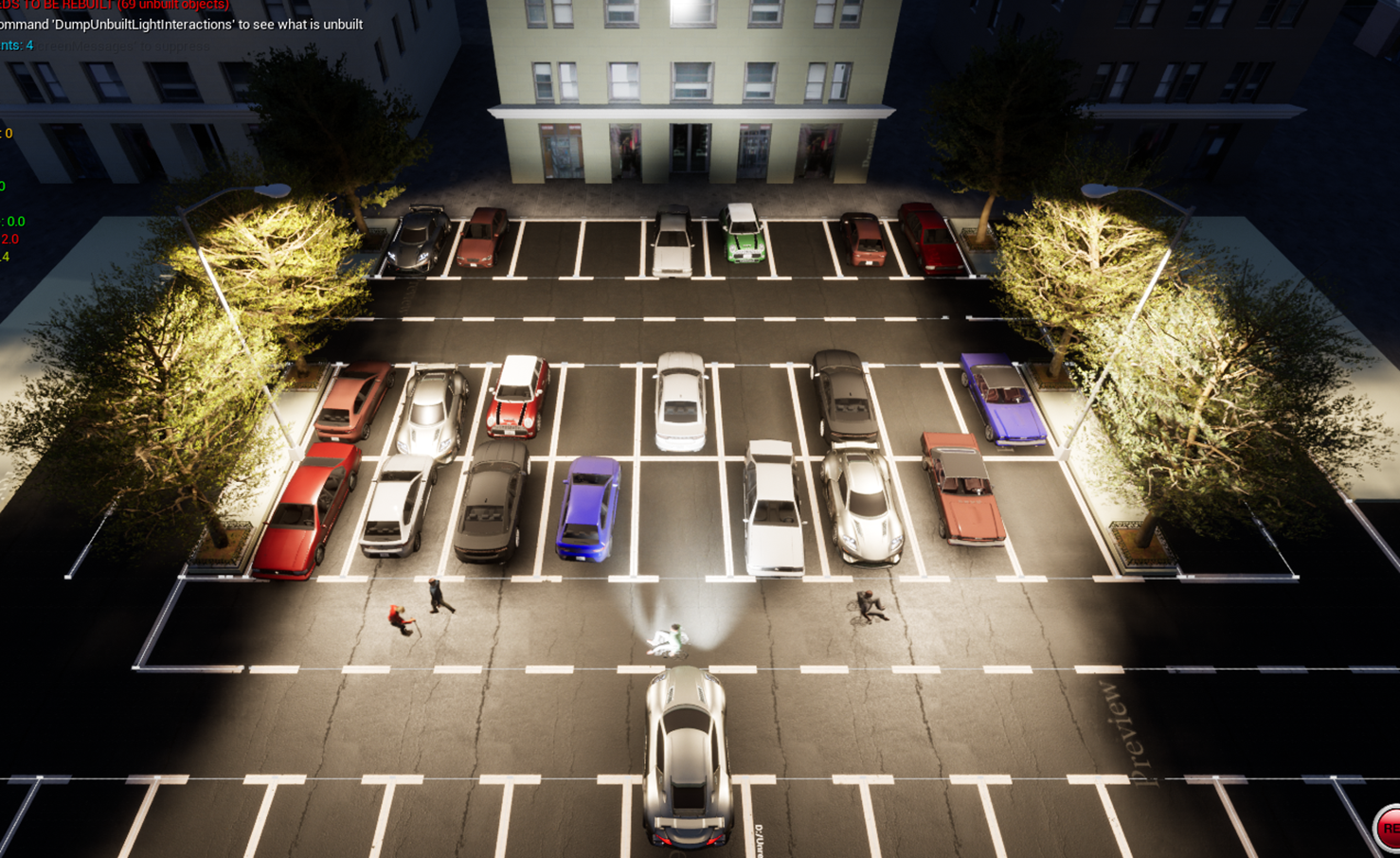
One of the critical challenges in autonomous driving and AI-based systems is the lack of diverse and representative datasets. Existing datasets often fail to reflect the broad range of pedestrians found in real-world scenarios—especially underrepresented groups such as people with disabilities or individuals from various ethnic minorities. This gap in representation can lead to the development of AI systems that are not equipped to handle the full diversity of pedestrians, potentially compromising the safety and inclusivity of autonomous driving technologies.
At Vicomtech, we have developed DiverSim, a cutting-edge simulator to solve this issue by generating synthetic data for pedestrians with a strong focus on diversity and inclusion. Built on the Unreal Engine 5 platform, known for its photorealistic capabilities, and leveraging the AirSim simulator as its foundation, this tool creates balanced datasets of pedestrians. It simulates a wide variety of individuals, including equal proportions of genders, different ethnic minorities, and individuals with various disabilities such as those using wheelchairs, crutches, or white canes for visual impairments. This simulator ensures that no minority is underrepresented, thus providing a balanced dataset that avoids biases that are common in most openly available datasets.
Users can also configure various simulation parameters, including atmospheric conditions, time of day, and the proportion of pedestrian types in specific scenarios. To ensure that the dataset is also visually diverse, some elements of the simulation are randomised each time it is executed, such as the size and shape of the background buildings, the position of cars and angle of the sun. The system supports two key use cases: crosswalks and parking areas. A customizable fisheye camera model is also integrated, a feature often missing in other simulators despite its prevalence in ADAS (Advanced Driver Assistance Systems). All generated data is annotated in ASAM OpenLABEL format.
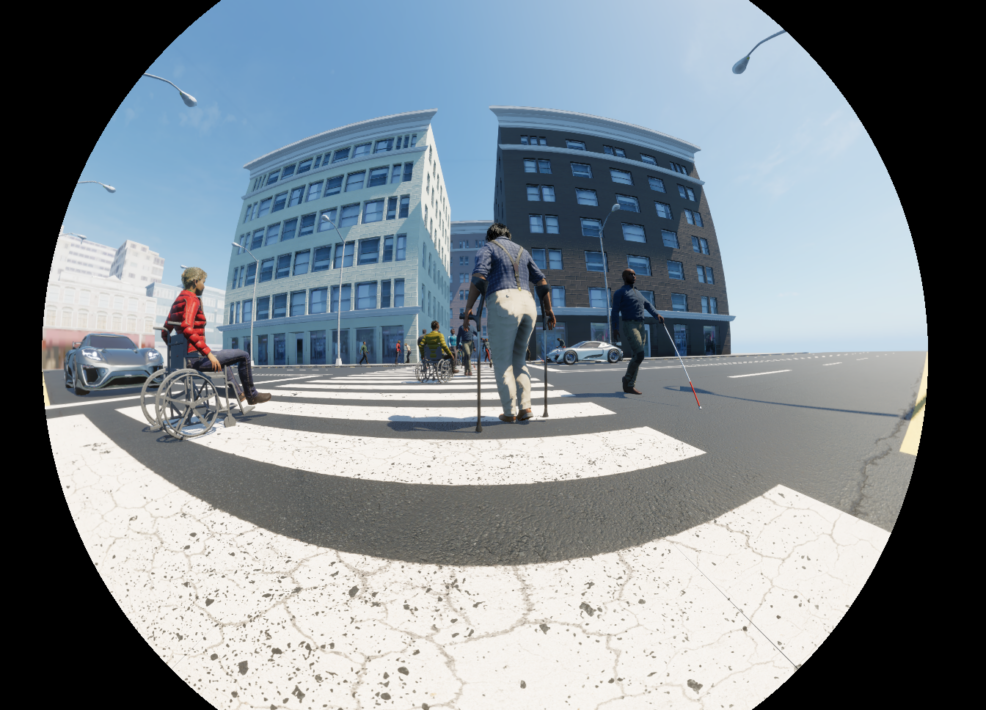
Moreover, the simulator and its data generation tools are now open source, allowing researchers and developers worldwide to use them freely. All the assets and animations used in this simulator have been carefully chosen so their licenses allow their use for AI training and validation purposes. This means that the data generated is readily usable for training and validating AI models designed to handle a wide range of pedestrian types and scenarios.
As future work, we aim to expand the simulator's capabilities by introducing 3D annotations and LiDAR sensor recordings, which will enable even more detailed data generation. We also plan to add additional use cases, like the VRU pick-up scenario, further enhancing the diversity of pedestrian interactions that can be simulated.
The simulator is designed to contribute to addressing dataset representation issues within the AWARE2ALL project, which aims to develop inclusive HMI (Human-Machine Interface) and safety systems for autonomous vehicles. By generating diverse, balanced datasets, the data produced will assist in re-training and validating AI models to better accommodate the needs of diverse individuals. This effort is integral to enhancing the safety and reliability of autonomous driving technologies for all users.
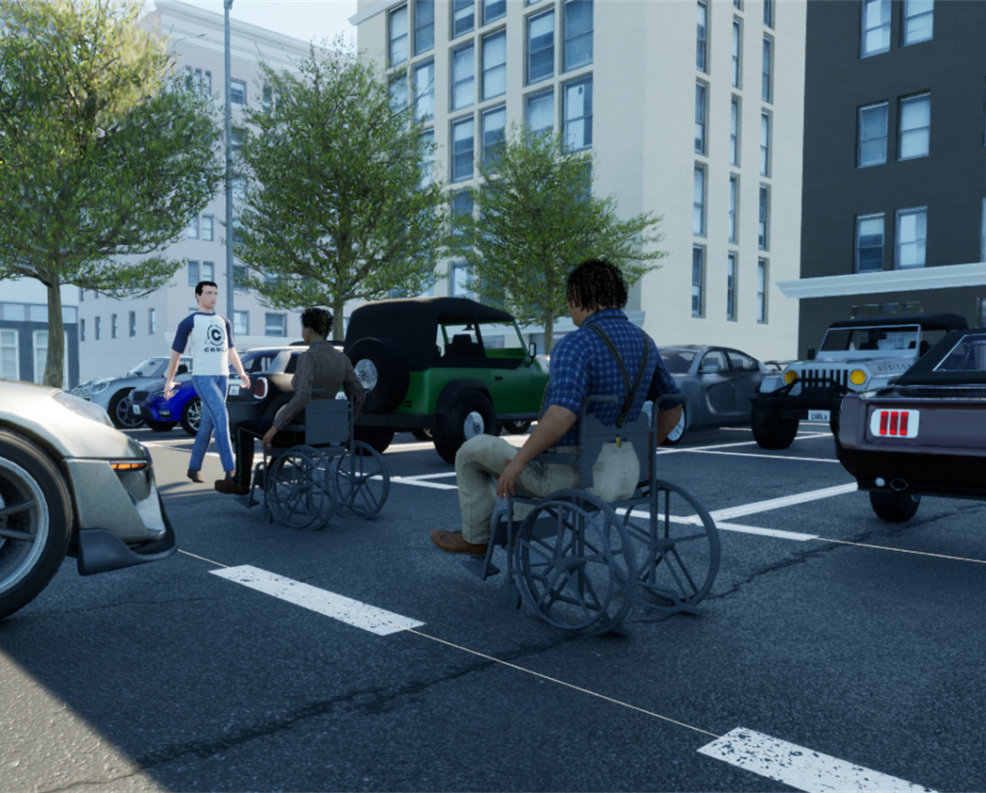
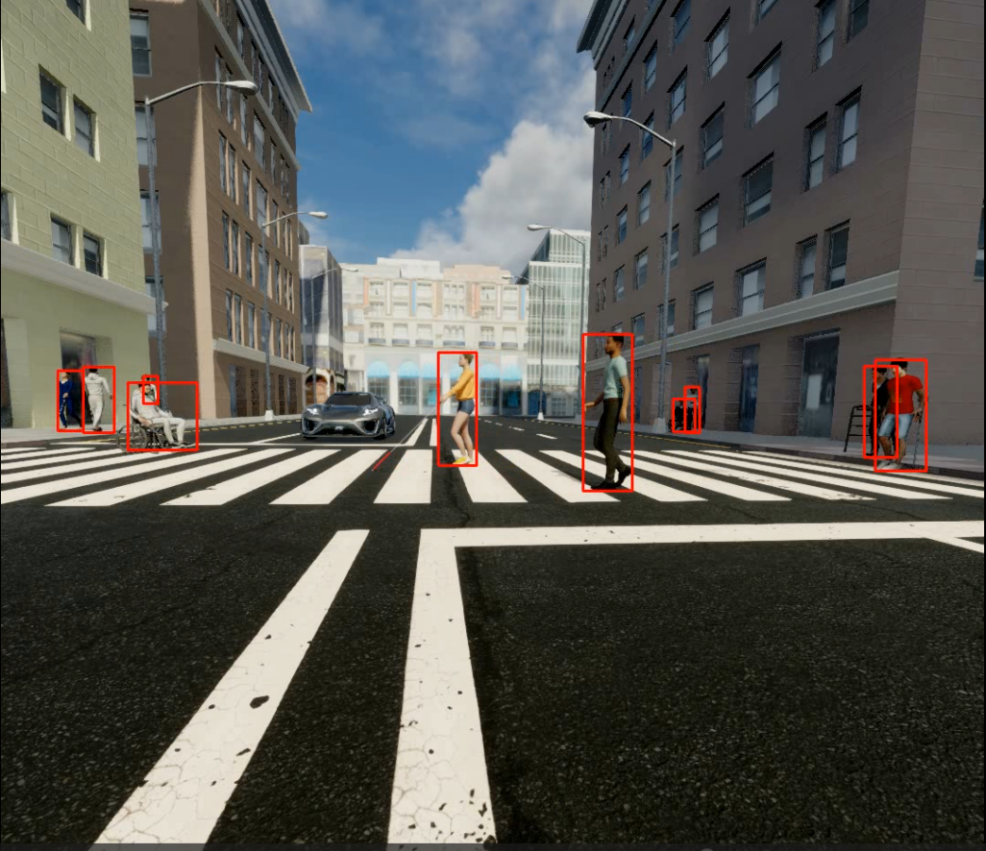